The World’s First, 97% Energy Reduction with Advanced Computing Performance
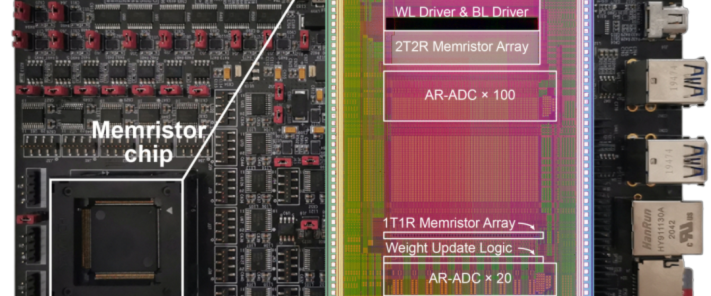
Computers are remarkable machines capable of performing a wide range of tasks, including gaming, web browsing, and problem-solving. However, computers face a significant challenge of their own: they often suffer from sluggishness and inefficiency when it comes to handling and processing data.
Data serves as the vital information that computers utilize to carry out their tasks, encompassing numbers, words, images, and sounds. This data is dispersed across various locations both within and outside the computer, such as disks, memory, and cloud servers. Whenever the computer needs to access specific data, it must transfer it from one location to another, resulting in time consumption and energy expenditure. This can be likened to having to visit the library every time you wish to read a book.
The need of sending data back and forth also consume a huge amount of energy. By contrast, it is estimated that a human brain uses roughly 20 Watts to work – that is equivalent to the energy consumption of your computer monitor alone, in sleep mode.
To address this predicament, scientists once again (after the invention of artificial neural network) look to human brain for inspiration. As a result, the idea of computing-in-memory has emerged as a novel technique capable of enhancing the speed and efficiency of computers by circumventing this issue. The concept revolves around storing and processing data entirely within the memory, which is a type of storage situated in close proximity to the processor—the component responsible for carrying out calculations. In essence, this is comparable to having the book directly in your hands, enabling you to read it instantaneously without any delays.
Computing-in-memory has unique advantages when it comes to artificial intelligence. Current technologies for training neural networks require moving massive amounts of data between computing and memory units, which hinders the implementation of learning on devices. The issue becomes even more pronounced with the emergence of large language models. The colossal parameters accompanying these models have resulted in a relentless surge in computational power demands. Training one LLM can use as much electricity as over 1,000 U.S. households in a year, and have a similar carbon footprint as five average cars. The energy consumption and environmental impact of LLMs depend on the model size, which determines the amount of computing power and cooling required. Larger and deeper models are usually more accurate, but also more costly to train and run.
The need to bridge this substantial computational power gap and achieve substantial enhancements in energy efficiency has become increasingly pressing. That’s when scientists from Beijing’s Tsinghua University turn to the idea of computing in memory with the innovative design of memory resistor (memristor).
A memristor is a type of electrical component that can remember how much current has passed through it. It can change its resistance, which is how much it resists the flow of current, depending on the previous current. This means that a memristor can store information without needing power, unlike other types of memory devices.
To understand how it works, think about a water faucet. A water faucet controls the flow of water, just like a resistor controls the flow of current. A normal faucet has a fixed resistance, which means that it always lets the same amount of water through when you turn it on. A memristor is like a faucet that can change its resistance, depending on how much water has flowed through it before. For example, if you let a lot of water flow through the faucet, it will become easier to turn on and let more water through. If you let only a little water flow through the faucet, it will become harder to turn on and let less water through. The faucet will remember how much water has flowed through it, even if you turn it off. This way, the faucet can store information about the water flow.
The memory resistor computing-in-memory technology is a new approach that transforms the traditional way computers work. It makes significant improvements in both computational power and energy efficiency. This technology takes advantage of the unique learning capabilities of specific components in the computer to support real-time learning directly on the computer chip itself. This empowers the computer to learn and adapt quickly, especially in situations where it needs to learn from its surroundings.
Currently, researchers are mainly focused on demonstrating how this technology can be used to improve learning using arrays of memory resistors. However, there are still challenges in creating fully integrated computer chips that effectively support on-chip learning. One of the main challenges is that the traditional method used for updating the weights in the learning algorithm does not work well with the actual characteristics of the memory resistors.
To tackle these challenges, the research team has come up with a new algorithm and architecture called STELLAR (STorage-Embedded Low-Latency Adaptive Reconfigurable). It is specifically designed to enable efficient on-chip learning by integrating memory resistors into the computer chip. Through innovative approaches in algorithms, architectures, and integration methods, the team has successfully developed the world’s first fully integrated memory resistor computing-in-memory chip that supports efficient on-chip learning. This chip includes all the necessary circuit modules to enable complete on-chip learning.
Edge learning using a fully integrated neuro-inspired memristor chip
The chip has been tested and shown its capabilities in various learning tasks such as image classification, speech recognition, and control tasks. It has demonstrated high adaptability, energy efficiency, versatility, and accuracy. In fact, it consumes only 3% of the energy compared to dedicated integrated circuits (ASIC) systems using advanced processes when performing the same tasks. This highlights its exceptional energy efficiency advantages.
The world‘s first fully integrated memory resistor computing-in-memory chip and test system.
This technology has the potential to meet the high computational power requirements of the AI era and provides an innovative path to overcome the energy efficiency limitations of the traditional computer architecture. The research findings, titled “Edge Learning Using a Fully Integrated Neuro-Inspired Memristor Chip,” were recently published in the journal Science.