AIGC and Beyond – The Road to Artificial General Intelligence
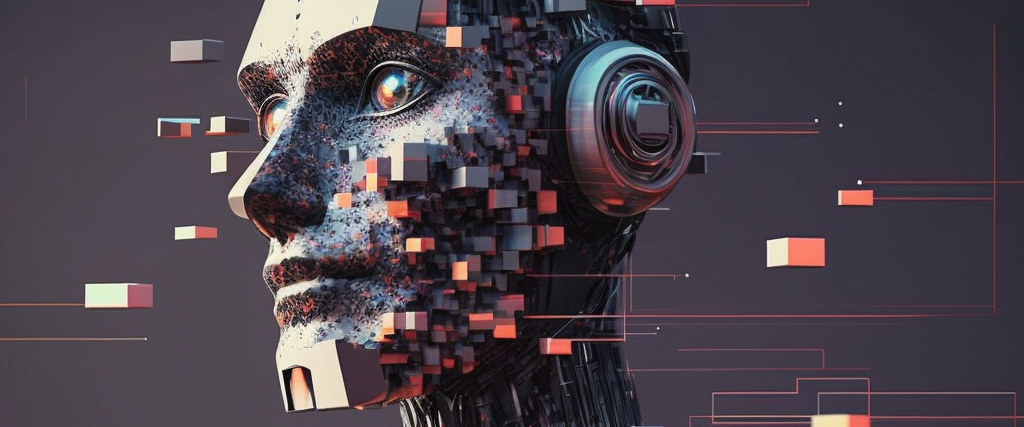
Last time, we discussed ChatGPT, an AI language model taking the world by storm. Today, we’re diving even deeper into the potential of AI in robotics.
In a recent article, we detailed the progress of the Google and SJTU teams in this field. And these are the main points you may need to know:
Until now, we have discussed situations when robots and AIs work alone as individuals, but in some cases, multiple AI or intelligent agents need to work together for a very complicated mission. Now consider this:
Here’s a team of robots, each with its unique abilities and programming. Now they faced their greatest challenge– to search and rescue earthquake victims buried in the rubble. But as they began to move, something was off. The sleek and agile robot hesitated at the designated waypoint while the hulking behemoth’s movements threw off the team’s maneuvers. Chaos erupted as the robots struggled to compensate, colliding and losing balance. They failed to understand each other, and the mission seemed doomed to fail.
Fortunately, it is just an exercise, and no real victims are waiting to be rescued. But the chaos illustrates the need for cooperation among these robots.
Enter the Multi-Agent Transformer (MAT) architecture. Again, it’s based on the transformer architecture we introduced in the previous episode. In this case, the transformer is modified and specialized for Multi-Agent Reinforcement Learning (MARL) to train the AIs for cooperation. It is proposed by the same group of researchers from Shanghai Jiaotong University, this time jointly with partners from Peking University and other Chinese scientists working in the UK.
MAT can help multiple agents, like robots or game players, work together to achieve a common goal. It does this by taking in information from each agent about what they see and know and then using that information to help each agent make better decisions. Think of it like a group of friends playing a game of soccer. Each player has their perspective on the field, but they need to work together to score goals and win the game. MAT would help each player understand what the others are doing and use that information to make better decisions about where to move and how to pass the ball.
MAT does this by using a special type of computer program called an encoder-decoder architecture. The encoder takes in information from each agent and turns it into a set of hidden states for ease of processing, which are then used by the decoder to generate actions for each agent. This process is repeated over and over again until the agents achieve their goals.
The summary of dataflow in MAT
Essentially, the MAT architecture works like having a coach in a football game who can help each player make better decisions by analyzing the movements of all players on the field. The coach can see everything that’s happening and guide each player based on what they see.
Multi-Agent Sequential Decision Paradigm: Conventional multi-agent learning paradigm (left) wherein all agents take actions simultaneously vs. the multi-agent sequential decision paradigm (right) where agents take actions by following a sequential order, each agent accounts for decisions from preceding agents as red arrows suggest.
The researchers test the performance of MAT in computer simulations. They used MAT to train agents to work together to complete tasks such as pushing a block or balancing a pole. The results showed that MAT outperformed several other algorithms in terms of both learning speed and final performance. They also found that MAT was able to improve continuously during training.
One of super-hard scenarios are shown below.
With this new algorithm, we probably won’t see the chaotic operation shown earlier. Multiple robots can be controlled by one AI model and act like one person. However, this approach is not without its limitations and challenges. For one thing, if the number of robots increases, their possible states and actions grow exponentially. This can make it difficult to model the joint strategy of all robots using this approach. For another, the differences in the capabilities or characteristics of different robots could also add to the complexity. For example, some robots may be better at certain tasks than others or have different goals. It’s hard to design a single approach that works well for all robots.
Future research may have to address these potential issues. Up to this point, we can see these scientists have been actively tinkering and testing the transformer architecture for a variety of applications. These efforts may one day extend the influence of AI beyond the virtual world and into the physical realm. But the scientists and entrepreneurs in China certainly won’t satisfy with this and will stop here. In the next episode, we will peep into the future – that’s a city inhabited totally by AIs, and we will see how the AI society will evolve.