After ChatGPT & AIGC, AIGA (Action) Might Be the Next Big Thing
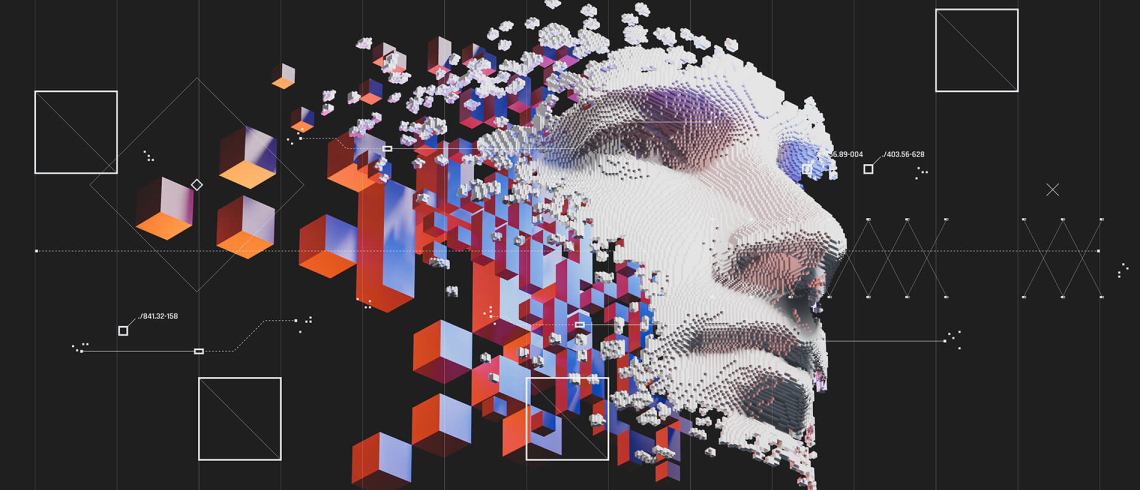
AI’s ability to generate high-quality content, or AIGC (AI-generated content), as demonstrated by ChatGPT, has taken the world by storm. The team behind ChatGPT also released research showed the use of language models to control robots. This development suggests that the influence of large language models could extend beyond the virtual world and into the physical realm. Naturally, they are not the only ones exploring this idea.
Last month, Google shared their solution, a multimodal language model called PaLM-E. PaLM-E can perform embodied reasoning tasks, meaning it can understand physical environments and objects. It can also perform visual-language tasks, like answering questions about pictures, and operate on multimodal sentences. Even more remarkably, PaLM-E can transfer knowledge from visual-language domains to embodied reasoning tasks, allowing it to learn from what it sees and improve its understanding of the world. One of its abilities is predicting affordances, or determining whether a certain action can be performed in a given environment.
For example, it can figure out if a robot can pick something up in a room without knocking anything over. PaLM-E is undeniably a significant breakthrough in AI that could pave the way for new applications in robotics. However, it’s worth noting that the demonstration video was shot in a lab environment, without much uncertainty, and the task is relatively straightforward.
PaLM-E controls a mobile robot operating in a kitchen environment. Up: The final steps of executing a plan to retrieve a previously unseen block (green star). This capability is facilitated by transfer learning from the vision and language models. Down: The task is to get a chip bag. PaLM-E shows robustness against adversarial disturbances, such as putting the chip bag back into the drawer.
Relying solely on language models for reasoning and decision-making has inherent disadvantages when it comes to controlling robots operating in real-life environments. Language models are capable of making decisions and reasoning to a certain extent, but they are not fully autonomous decision-making systems. They are trained on vast amounts of text data and use statistical patterns to generate responses to input. While they can generate seemingly coherent and relevant responses, they do not have a true understanding of the meaning behind the words they use.
Thus, language models can make decisions and reason based on patterns learned from their training data, but they cannot reason or make decisions like humans. They are limited by the data they have been trained on and the algorithms used to generate responses. So, if language models are not enough, what would be needed to add to the mix?
Recently, a joint research team from Shanghai Jiaotong University and Shanghai Digital Brain Laboratory released a video demonstrating a quadrupedal robot walking on various terrains and adjusting the length of its limbs and torso to accommodate the ups and downs of the terrain. For example, it can extend its hind legs longer by elongating its “tibias” when climbing up a slope and do the reverse when climbing down. The robot can do this smoothly, without the hesitation typically seen in robots during such transitions. It doesn’t need to be told what to do; instead, it can analyze the terrain and plan its next moves and adjust its body as necessary.
While this may not seem as impressive as parkour-practicing or acrobatic robots, the way this robot is controlled and its awareness of both the environment and its own body state are truly noteworthy. In this demonstration, instead of using large language model to control the robot, as in the case of OpenAI / Microsoft (and many other teams, such as DeepMind / Google, for that matter), the Shanghai team utilizes the Foundation Decision Model to realize what they call AIGA, that is, AI-generated actions. The Foundation Decision Model (FDM) has a lot in common with large language models (LLM). For one, it’s also based on the Transformer algorithm. Transformer is predominantly used in processing natural languages, using its unique ability to process input in parallel using an attention mechanism. This is like having a team of translators/summarizers working on different parts of the input at the same time, rather than a single person going through the input step-by-step. The attention mechanism is like a spotlight, highlighting the most relevant parts of the input. The Transformer learns where to focus the spotlight to generate the best output. And here, rather than using it to process sentences, which are a sequence of words, the Shanghai team want to use it to deal with sequence of moves, with a series of decisions to make.
The other similarities between FDM and LLM include they are both pretrained model that are trained offline and can be used later upon the requests of humans for varying tasks, and from the feedback of humans they can be improved. This puts them in stark contrast with what now to be known as classic machine learning, in which a specific task, such as facial recognition task, need to be defined in advance before the compiling of dataset and the training of model can be proceed. The large model or foundation models work the other way around. They are trained in advance on vast amount of data and the tasks are later assigned upon the request of users, as with ChatGPT.
The AIGA envisioned by the Shanghai team would integrate decision model and language model. Currently, to enable language models for tasks beyond generating texts, such as doing complex scientific calculations, the current practice is to connect the model with various APIs that provide specialized tools. This approach works well except one thing: there should be a suitable API available for the problems at hand. And we all know that in reality, things rarely go as predicted, and unexpected situations arise. In such scenarios, the model would need to be able to analyze the problem and find the solution by itself. A robot operating in a real environment is far more likely to encounter unexpected issues than a language model chatting with you through a browser.
For example, a quadrupedal robot might be instructed via the language model to go from point A to B but come across several obstacles and potholes along the way. That is where the decision model becomes invaluable for completing the task, as it helps the robot plan the route and navigate successfully. As it walks on, it may encounter different terrains, barriers or pedestrians in the way. These in turn generate feedbacks through the robot’s sensors, informing its “brain” (the two models) what’s going on in the environment. Sometimes the feedback doesn’t necessarily go to the language model; instead, it will go directly to the decision model, much like humans don’t keep telling ourselves “go left” or “go right” while we are walking. We don’t use the language areas in our brain for every physical move either. Using this paradigm, the team has trained robots to walk smoothly, not only in simulator programs on computers but also with fewer stumbles in real environments compared to robots controlled by other algorithms.
AIGA Paradigm 1 (Standard Scenario): Centered on the large language model, it cooperates with the decision-making Environment selected by APl; AIGA Paradigm 2 (Non-standard Scenario): Generalized Decision-Making Control Centered on Large Decision-Making Models
Decision model may be better equipped than language models in dealing with uncertainties when steering robots through a variety of real-world tasks. But we need to keep in mind that all the research discussed here is in its early stages. Currently, the Shanghai team, particularly the Digital Brain Lab, are actively promoting the applications of their models in diverse industries such as gaming, entertainment, retail, energy and telecommunication. In a world of reinforcement learning from human feedback (RLHF), this would eventually bring about continuous improvement. The wave of revolution powered by AIGA might be closer to us than we thought.
Wang, H., Yang, M. A., Wangdue, S., Lu, H., Chen, H., Li, L., Dong, G., Tsrin, T., Yuan, H., Fu, Q., & 27 others. (2023). Human genetic history on the Tibetan Plateau in the past 5100 years. Science Advances, 9(11), eadd5582. https://doi.org/10.1126/sciadv.add5582
Yang, J., Jin, Z. B., Chen, J., Qu, J. (2017). Genetic signatures of high-altitude adaptation in Tibetans. Proceedings of the National Academy of Sciences, 114(16), 4189-4194. https://doi.org/10.1073/pnas.1617042114