OpenAI's Sora pours 'cold water' on China's AI dreams?
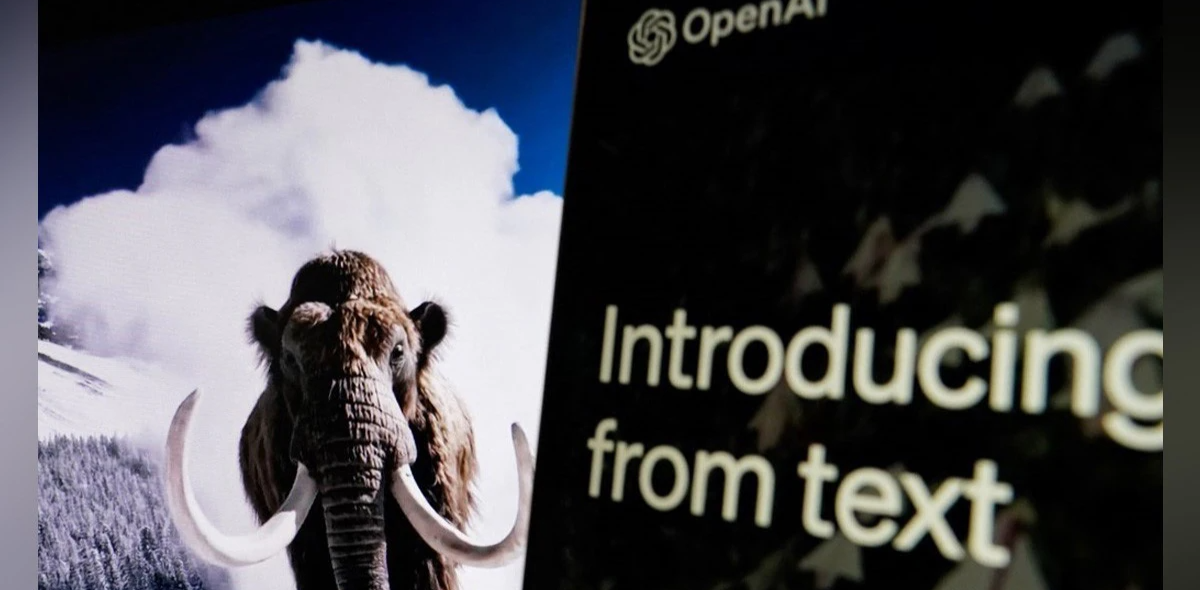
OpenAI’s SORA has made a significant splash worldwide, garnering immense attention for its ability to generate incredibly realistic videos of unprecedented length. However, amidst this buzz, there seems to be a relative calm in China’s AI landscape. Some commentators, both within and outside the country, hasten to claim that the AI gap between the United States and China is widening. Yet, what they fail to recognize is the quiet but powerful productivity revolution fueled by large AI models that is currently underway on this side of the Pacific.
While SORA has undoubtedly captured global headlines, it is important not to overlook the remarkable advancements taking place in China’s AI sector.
Behind ChatGPT lies around 30,000 GPU chips providing computational power, but Sora’s demand for computing power is even more staggering. Sam Altman, OpenAI CEO, is now aggressively pushing a $5 to $7 trillion chip plan to increase the supply of self-developed chips. However, $7 trillion is almost equivalent to two years of the UK’s GDP, making such an investment unrealistic for most countries. Pouring enormous sums of money into an arms race for computing power may not be the only path for the development of artificial intelligence.
In addition to developing general large models that rely on computing power and hardware, people are also constantly exploring the development of specialized large models for specific fields. These models require specialized training corpora like those used for industrial robots, intelligent driving, and weather forecasting. They require less money than general large models and can be directly applied in the industrial field.
Smarter robot worker
Recently, researchers from Tsinghua University, with co-workers from the Beijing-based Samsung Research China, have successfully trained a robot to comprehend and execute natural language commands. This innovative approach, known as RobotGPT, aims to improve the stability and safety of robotic manipulation tasks, making it more applicable to everyday life scenarios. The researchers are exploring the potential of integrating large language modules, like ChatGPT, into robotics systems, allowing robots to understand and respond to natural language instructions.
While ChatGPT can generate code for executing tasks, there is a risk of unpredictability and inconsistency in its outputs. This makes it challenging to directly integrate ChatGPT into the robot’s decision-making process.
To overcome this limitation, the researchers developed a robust learning model within the RobotGPT framework. The system translates the environment and tasks into natural language prompts, allowing ChatGPT to generate specific action command codes. However, instead of directly executing this code, RobotGPT learns the planning strategies generated by ChatGPT. This approach significantly improves the stability of the system and enhances task success rates. Although ChatGPT is used in this research, the framework can be easily applied to other similar language models, especially those trained by Chinese researchers with comparable coding abilities.
The combination of AI and robotics will eventually reshape manufacturing. In February, the Shenzhen-based Ubitech, also the world’s first humanoid robot producer ever went public, has its latest mode, Walker S, deployed in an EV plant. The robot’s “internship” encompasses a range of aspects, including adaptive walking for starting and stopping the production line, robust mileage calculation and walking planning, autonomous operation perception, system data communication, and task scheduling.
Ubitech has specifically developed the humanoid robot Walker S for industrial purposes and established partnerships with several prominent EV enterprises. In June of the previous year, Ubtech joined hands with Jiangsu-based Miracle Automation Engineering, a leading player in the manufacturing and supply chain of EVs, to establish a joint venture. This collaboration focuses on the research, development, manufacturing, and comprehensive solutions of humanoid robots tailored for the automotive sector. As a result of this partnership, Ubtech now stands as the sole humanoid robot company actively involved in the automotive industry despite not manufacturing vehicles themselves.
Expert models
In addition to manufacturing, Chinese AI models have been applied in many other highly specialized areas. HUAWEI’s Pangu is a case in point as they set their sights on one of the most challenging predictive tasks ever: weather forecasting.
The current method for weather prediction, known as numerical weather prediction (NWP), involves complex mathematical calculations that are computationally expensive and time-consuming. However, the new AI-based approach presents a more efficient alternative.
It uses deep learning and neural networks to understand how current weather conditions relate to future weather patterns. By studying a huge amount of global weather data from the past 39 years, Pangu-Weather can make very accurate predictions for medium-range weather.
What makes Pangu-Weather special is that it uses three-dimensional networks and special knowledge about Earth. It looks at the height of the atmosphere as a separate thing and can understand complex weather patterns at different levels. This makes its predictions much better than the old two-dimensional models.
Pangu-Weather also has a smart way of training its models. It trains a bunch of models with different forecast times, and this helps it make faster and more reliable predictions for medium-range weather. When tested against the best traditional weather prediction system, Pangu-Weather consistently gave more accurate forecasts.
The model also demonstrates an exceptional ability to track tropical cyclones. Pangu successfully tracked 88 tropical cyclones in 2018, including some that were difficult to track for other systems like ECMWF-HRES. For these 88 tropical cyclones, Pangu Weather’s tracking results were more accurate than the traditional ECMWF-HRES system. In terms of the average position error of the cyclone eye over 3 days and 5 days, Pangu Weather reported 120.29 km and 195.65 km, respectively, which were significantly smaller than the reported values of 162.28 km and 272.10 km by ECMWF-HRES. The advantage of Pangu Weather becomes more evident as the forecast lead time increases.
For example, when tracking the two strongest cyclones, Kong-rey and Yutu, in the western Pacific in 2018, Pangu Weather correctly predicted Yutu’s path (towards the Philippines) at 12:00 UTC on October 23, 2018, while ECMWF-HRES predicted a significant northeastward turn for Yutu, and it wasn’t until two days later that it predicted the correct path. Pangu Weather’s exceptional performance has been widely recognized. China’s Central Meteorological Bureau stated that Pangu Weather accurately predicted the trajectory of Typhoon “Mangkhut” in May, forecasting its eastward turn in the waters east of Taiwan Island five days in advance.
And this is just the tip of the iceberg of what Pangu is capable of. In fact, Pangu is the collective name of 5 large foundational models trained by HUAWEI, which include the Chinese language model, vision model, multi-modular model, scientific computing model, and graph model.
The Chinese Language (NLP) model is the first pre-training model in the industry with over 100 billion parameters, specifically designed for the Chinese language. It is widely regarded as the AI model that comes closest to the human-like understanding of Chinese. In comparison to ChatGPT, the Huawei Pangu model places greater emphasis on optimizing for the intricacies of the Chinese language.
The Visual (CV) model is the biggest CV model in the industry, with over 3 billion parameters. It has some exciting features, like on-demand model extraction and a balanced approach to understanding and generating information. This means it can adapt and be used quickly for different AI applications. By using special algorithms, the CV model can better understand images based on their features and improve its ability to learn from small amounts of data. It has become a top model in the industry.
On top of these 5 foundation models, HUAWEI has already launched industry-specific models like Pangu Financial, Pangu Mining, Pangu Pharmaceutical Molecular, Pangu Power, Pangu Manufacturing Quality Inspection, and Pangu Weather, as mentioned earlier.
Shorten the training time
On another front, Chinese researchers are seeking to significantly shorten the training time of large models. Scientists from Peking University and Bytedance, the parent company of TikTok, have just unveiled MegaScale, a production system for training large language models (LLMs) at the scale of more than 10,000 GPUs. Using this system, they accomplished the training of a GPT-3-sized model (175B) within just 1.75 days, while it took almost one year for OpenAI to train the model.
Training Large Language Models (LLMs) at such a large scale presents new challenges related to efficiency and stability during the training process. To tackle these challenges, a comprehensive approach called “full-stack” is employed. This approach considers all aspects of the system, including both the algorithmic components (the mathematical processes used in the model) and the system components (the hardware and software infrastructure supporting the model). A set of diagnosis tools is developed to monitor system components and events, identify root causes, and create effective techniques to ensure the system can continue functioning correctly even when some of its components fail.
One Bit is all it takes
While there are still other researchers trying to overturn the underlying logic of large language models and address the bottleneck of limited computing power, especially against the backdrop of the US chip embargo. Researchers at the University of Chinese Academy of Sciences and Microsoft Research Asia have made a groundbreaking leap with the introduction of 1-bit Large Language Models. This new development, known as BitNet b1.58, has the potential to revolutionize the way language models are utilized, offering remarkable benefits in terms of performance and cost-effectiveness.
Large Language Models’ increasing size has presented challenges in terms of deployment and raised concerns about their environmental impact due to high energy consumption. In response to these concerns, researchers have been exploring ways to reduce the computational and memory requirements of these models.
The BitNet b1.58 model represents an exciting breakthrough in this pursuit. Unlike traditional LLMs, which typically employ 16-bit floating-point values, BitNet b1.58 utilizes ternary values of {-1, 0, 1}, resulting in a 1.58-bit model. Despite the reduced precision, this new model matches the performance of full-precision models with the same size and training tokens, as measured by perplexity and end-task performance.
The advantages of BitNet b1.58 are manifold. Firstly, this model offers significant cost savings in terms of latency, memory, throughput, and energy consumption. By employing integer addition instead of floating-point operations, the new computation paradigm of BitNet b1.58 achieves substantial energy savings and faster computation, making it highly efficient.
BitNet b1.58 addresses the issue of transferring the information learned by an AI model from external storage to specialized hardware that accelerates the processing of AI models. By using less memory space, BitNet b1.58 reduces the time and cost associated with loading these model parameters, resulting in a faster and more efficient process of using the AI model to make predictions.
BitNet b1.58 also introduces explicit support for feature filtering, which means it can better analyze and select important features in the data. This enhancement improves its ability to create accurate models and leads to better performance in AI tasks.
In experiments, BitNet b1.58 has shown that it can perform as well as models that use standard, high-precision numbers for calculations starting from a model with 3 billion parameters when using the same configuration. This means that even with its optimizations and reduced memory usage, BitNet b1.58 can still deliver the same level of performance as larger, more resource-intensive models.
The implications of this research extend beyond improved language models. The introduction of 1-bit LLMs opens the door for designing specialized hardware optimized for these models. This novel approach has the potential to redefine the future of AI hardware and pave the way for even more advanced and cost-effective language models.
Therefore, let’s revisit the question posed in the title of this article: has the AI gap between China and the US widened after the arrival of SORA? It’s important to remember that when measuring progress in AI, video generation is far from the only game in town. At present, Chinese models may not be able to create videos of comparable quality to SORA, but there are numerous other fields where the advancements of Chinese models are unmatched.
China’s “industry-specific large models” have been applied to mining, meteorology, medicine, and electric power. As the world’s most powerful manufacturing country in history, this is the important logic and unique advantage of China’s development in artificial intelligence.
More importantly, behind all these AI developments, including SORA itself, are contributions from researchers of various nationalities, including both the US and China. This highlights the true nature of scientific research, which thrives on the sharing of knowledge and collaborations that transcend national borders.