Bypassing the GPU Blockade: China’s Taichi II Microchip is Coming
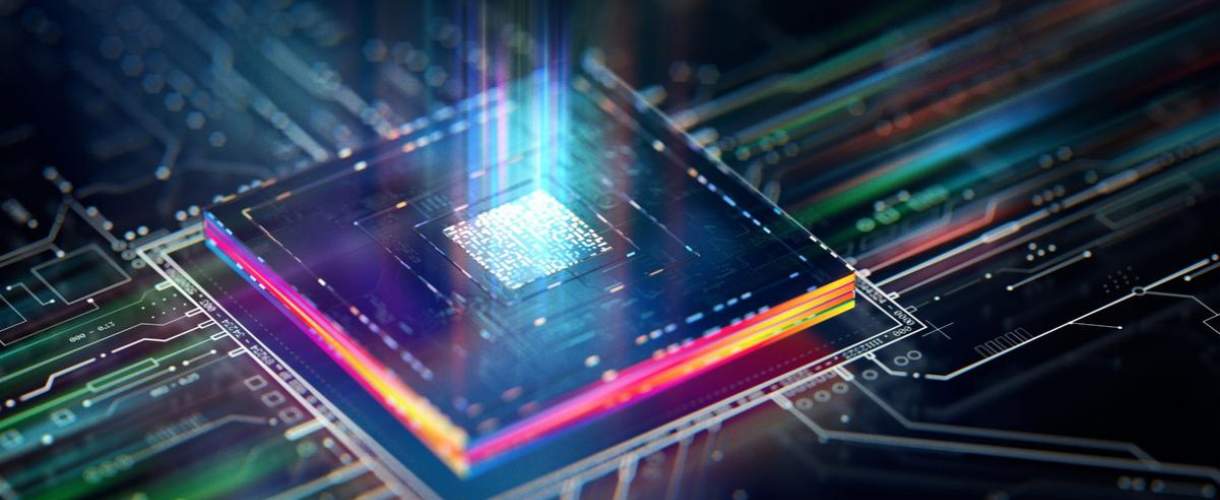
The US government has been tightening the export control of high-performance GPU as well as placing sanctions on Chinese tech firms, in an effort to slow down China’s progress in AI. However, these flawed and porous measures are doomed to fail.
Scientists from China’s Tsinghua University have developed a revolutionary new method for training optical neural networks, potentially paving the way for faster and more energy-efficient artificial intelligence systems that don’t rely on GPUs at all. The research, published in Nature on recently, introduces a technique called “fully forward mode” (FFM) learning, which allows for on-site training of optical systems without the need for complex computer simulations.
Optical computing, which uses light instead of electricity to process information, has long been touted as a promising alternative to traditional electronic computers. Much like how fiber optic cables can transmit data faster than copper wires, optical computers have the potential to perform calculations at much higher speeds while consuming less energy.
However, training these optical systems has been a significant challenge. Previously, researchers had to rely on computer simulations to design and optimize optical neural networks, similar to how architects might use computer models to design a building before construction. This approach was often inaccurate and inefficient, as the simulations couldn’t perfectly account for real-world imperfections in the optical systems.
In addition, in contrast to model reasoning (using AI models to perform tasks), model training demands substantial computational power. However, current optical neural network training heavily leans on GPUs for offline modeling and necessitates precise alignment of physical systems. As a result, the scale of optical training faces significant limitations, appearing as though the advantages of optical high-performance computing are confined by intangible constraints.
The new FFM learning method addresses this problem by allowing the optical system to learn and adjust itself in real-time, much like how a self-driving car might continuously adapt to road conditions. This on-site learning approach eliminates the need for complex simulations and allows the system to account for its own unique quirks and imperfections.
The Tsinghua researchers demonstrated the power of their new technique through several impressive experiments. First, they created a deep optical neural network capable of recognizing images with accuracy comparable to traditional computer-based systems. This is akin to teaching a camera to recognize objects as well as a human can, but using only optical components.
Subsequently, the team developed an imaging system that could see through scattering materials (like fog or frosted glass) with unprecedented clarity. This technology could potentially be used to improve visibility for self-driving cars in poor weather conditions or enhance medical imaging techniques.
Moreover, they also demonstrated an “all-optical” system capable of processing information using extremely low light levels – equivalent to less than one photon per pixel.
And finally, the researchers were able to design an optical microchip based on this FFM learning method, known as Taichi II, with the hope to replace GPU currently used and significantly improve the training efficiency of AI systems.
The implications of this research are far-reaching. Optical AI systems could potentially process information much faster and more efficiently than current electronic computers, leading to advancements in fields such as autonomous vehicles, medical diagnosis, and climate modeling.